No-/Low-Code data and AI platforms: what do they do? | Sharing the data workflow
The current discussion about the putative waning role of data scientists has to do with the technology that is available to non-data scientists. I am starting a multi-episode review of the platforms in this space. The first question is: what are the drivers of the data and AI workflows?
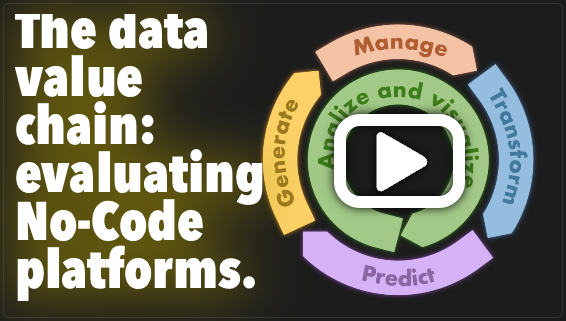
In the past few months, I'm sure we've all noticed the emergence of the Low-/No-code category in the marketing of tech. The idea of platforms making it possible for users to produce technology-driven artefacts without the skills to develop the tech themselves has been around for a while, especially in the website-creation category. But this capability seems to have expanded to a host of other use cases.
And of course, there's a marketing term for it. Two, actually: No-Code and Low-Code.
The terms are pretty self-explanatory, so I won't delve into defining them. Suffice it to say that they intend to put the users of tech closer to being able to build their own applications. It's however interesting to notice the appearance of the "Low-code" category name a little later than the "No-Code" one. It seems to turn out that, for the core user-facing workflow value, i.e. the part of the application that does very specific things for a certain category of users, the technology gap can be closed with a relatively small amount of code that is free of important system engineering considerations. Code that just does obvious simple things that a savvy user can figure out.
I think it's rather uncontroversial that in any development project that develops an application from scratch, a massive proportion of the time is spent on coding all sorts of back-end software that deals with deployment, security, pipelines, data management and so on, that a) is invisible to the user and b) is very likely functionally almost identical to other back-end software developed for different use-cases.
Also, a lot of the user-facing value creation work is really spent on trying to figure out, clarify and document exactly what needs to be done. It involves a lot of transfer of knowledge and information between the users / POs and the dev team, both before and after code has been (re)written, which represents a very large proportion of the team's time, rather than time spent writing working code.
Similar things could be said about the work of data scientists and data analysts in the context of building data applications.
As a general rule, I'm very focused on the question of removing artificial or obsolete boundaries between teams and the delivery of value, so I find the promise of having platforms that enable the users to focus on their expert business data needs and be able to configure data pipelines themselves, or with anecdotal engineering support, quite enticing.
I have therefore decided to get to understand these platforms better so as to qualify the opportunity that they represent. I started to review and test a variety of data-related platforms with real data. I will share my findings on an ongoing basis, and am using an analysis framework that takes the data value-chain as a basis. In this video, I explain what that simple framework is, and I will publish platform- and capability-specific video reviews going forward.
Note that there are three major aspects that are not covered by this simple model:
- NLP-related considerations,
- Data modeling, which is a critical missing part,
- The decision-support applications themselves.
I will, if I have time, deal with these separately, and hopefully gradually build up to a comprehensive view.
Do you have experience in No-/Low-code data and AI platforms? Do you suggest that I also look at platforms that I don't mention in this video? If that's the case, then let me know and I'll put them on my list to do a review.
You can subscribe below to receive these updates as a newsletter and comment on this platform. If you prefer to engage on LinkedIn, that's fine too.
Comments